Quick Tips: Initial Universe, Multi-Account Strategies, .zshrc
Thu Dec 12 2024This article's tips:
- Quick Tip 1: Speed up Pipeline with
initial_universe
- Quick Tip 2: Trade strategies in multiple accounts
- Quick Tip 3: Store terminal shortcuts in a .zshrc file
Quick Tip 1: Speed up Pipeline with initial_universe
The Pipeline API is a powerful library for performing cross-sectional computations on the entire universe of US stocks. But what if you need to research just a few stocks? Using the screen
argument to filter the stocks you want isn’t ideal because Pipeline will compute values for all stocks then apply the filter afterwards, resulting in unnecessary computational load. A better approach is to use the initial_universe
argument (a QuantRocket enhancement to the Pipeline API), which drastically speeds up performance by applying the filter before computing values, avoiding excess computations.
Learn more about when to use initial_universe
and when to use screen
in the usage guide.
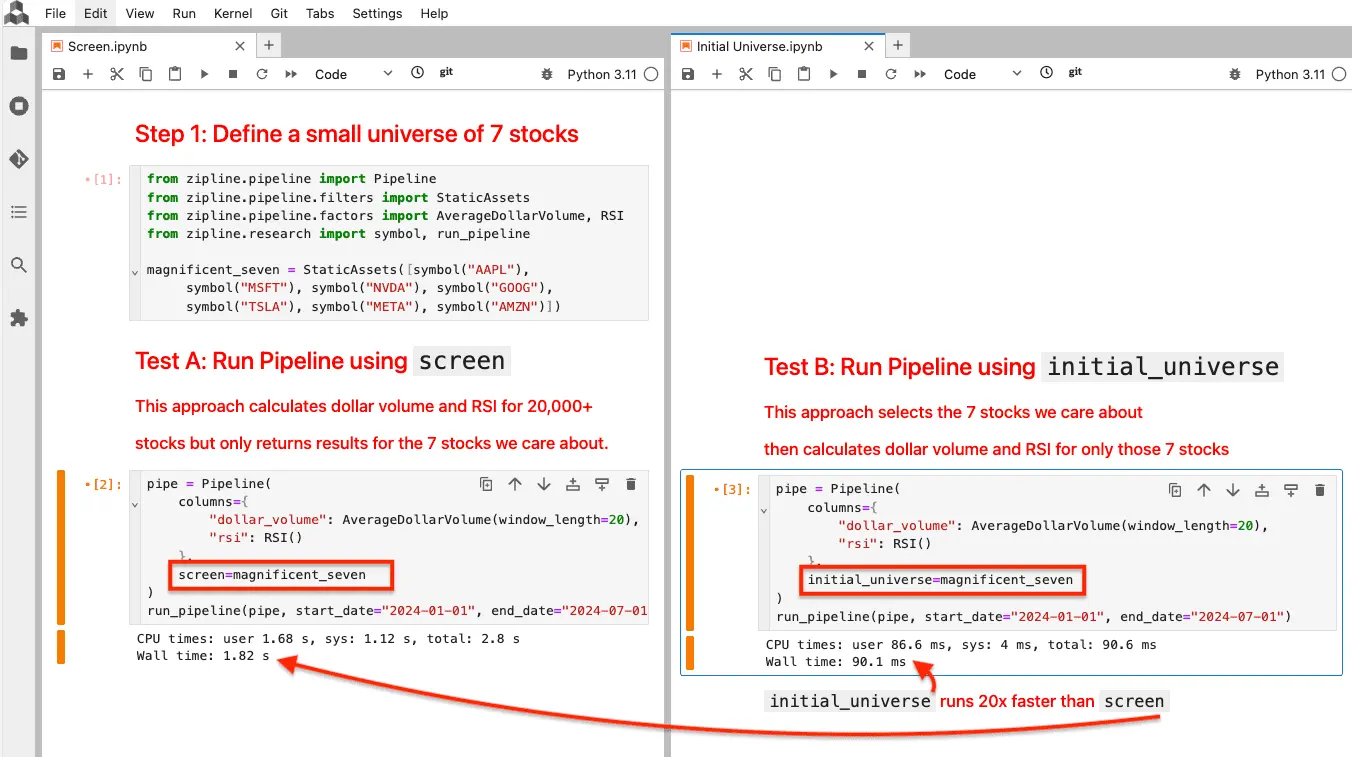
Quick Tip 2: Trade strategies in multiple accounts
If you're a trader with multiple accounts or a financial advisor managing multiple client accounts, QuantRocket makes it easy to run automated trading strategies in all of your accounts. Define how much capital to allocate in each account, and QuantRocket will generate a batch of account-specific orders. Alternatively, you can utilize FA orders, which split and allocate a single order placed to the master account across multiple sub-accounts based on user-defined allocation methods.
Learn more in the Financial Advisers section of the usage guide.
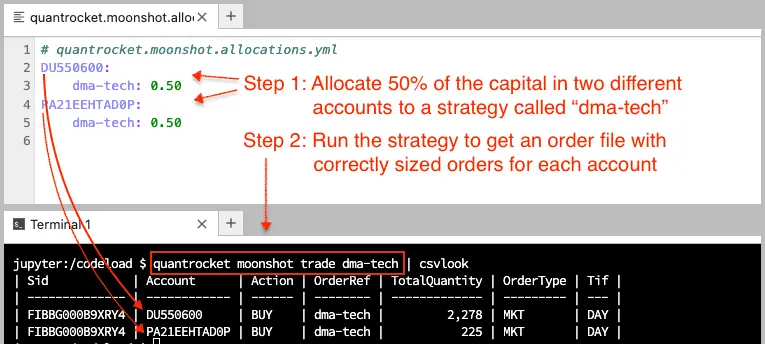
Quick Tip 3: Store terminal shortcuts in a .zshrc file
Create a .zshrc file in QuantRocket to define shortcuts for commonly used terminal commands.
In Linux, a .zshrc file is a hidden script file that runs each time you log into a terminal and is used to customize the terminal. In QuantRocket, you can create a .zshrc file from the JupyterLab Launcher and define shortcuts in the file using the Linux alias
command.
Shortcuts are great for common monitoring tasks such as monitoring your balance or portfolio or running custom monitoring scripts.
Learn more in the usage guide.
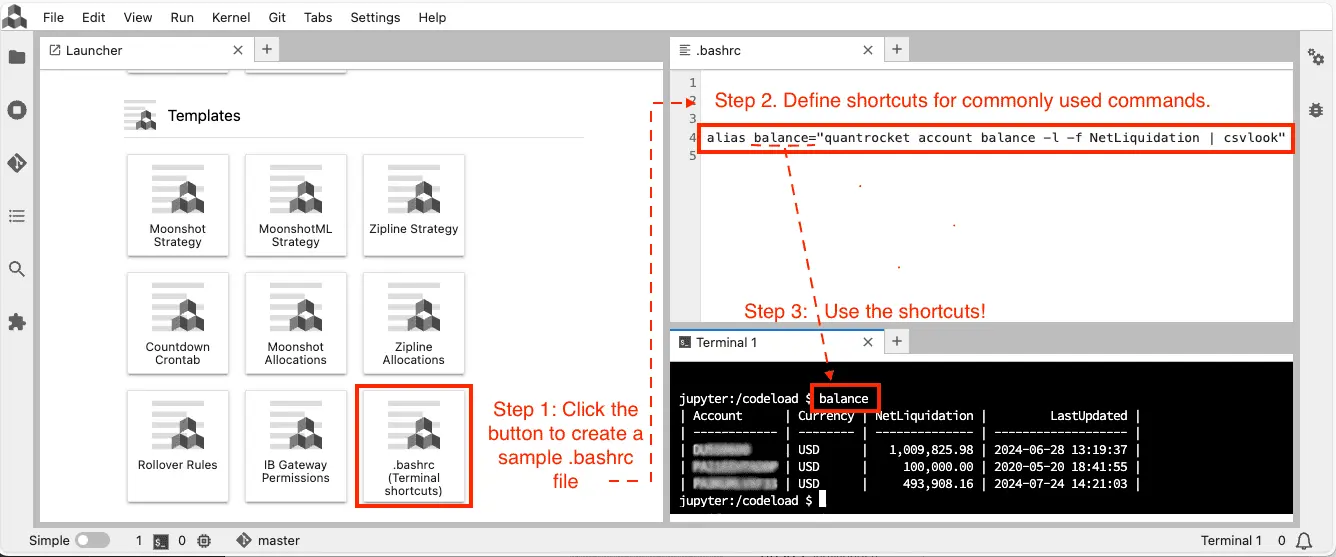
About QuantRocket
QuantRocket is a Python-based platform for researching, backtesting, and trading quantitative strategies. It provides a JupyterLab environment, offers a suite of data integrations, and supports multiple backtesters: Zipline, the open-source backtester that originally powered Quantopian; Alphalens, an alpha factor analysis library; Moonshot, a vectorized backtester based on pandas; and MoonshotML, a walk-forward machine learning backtester. Built on Docker, QuantRocket can be deployed locally or to the cloud and has an open architecture that is flexible and extensible.
Learn more or install QuantRocket now to get started.